Articles
Working with Imperfect Data
- By AFP Staff
- Published: 12/13/2022
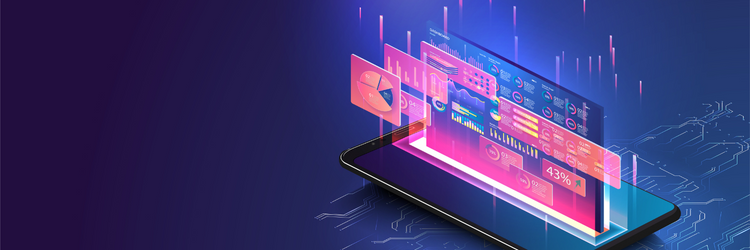
We are continuing our effort to help the finance community get their data right. We began in June with the first FP&A Series, continued with a guide in September, and now, with a recent webinar, we present points of view from outside finance on this important topic.
To help us do that, we enlisted the help of Najeeb Uddin, senior vice president and CIO at AARP, and Ed Cook, president of The Change Decision and consultant with the Association for Change Management Professionals. The following is part one of a two-part article (read part two here).
COMMUNICATE SHARED GOALS TO CREATE CONSISTENT DECISIONS
If you want to be successful, you have to be clear about the goals that the entire organization can support. To accomplish this, first, the shared goals need to be ones that everyone can support. “It's very hard to implement a shared system to four different goals,” said Uddin. Departments and business units have their own goals, but you have to come up with some version of shared goals to overcome silo mentalities.
Second, clarity starts with communication — communication, communication, and when you’re done, more communication. You have to communicate what you're doing — and you have to be okay with starting over. It's not a one-time process that you do in one pass and you're done. You learn, and then you re-clarify the goals which leads to more communication.
The common error is to look for a quick start to a data or technology project and bypass this step, but you will save time and money through this initial effort. “People want to blow by this part of the conversation,” said Uddin. “They want to get into the solution, they want to get into building stuff. They're already late, there's already stuff to do, but if you don't spend that time up front to have those conversations, put it down on paper, explain it over and over, you wind up paying for it on the tail end.”
Start off with the questions: What do you care about? What's going to be important to you as we figure out the shared goals we’re going to tackle together? And then, what actions are you actually going to take?
“The first thing I think about when we start deliberating about different sets of goals is getting people together and thinking,” said Cook. “What are we going after?” Ask people to articulate what they care about when you talk about “the goal.”
“Say the goal is to increase revenue. That makes sense, and we could talk about a new market, but why do you care about that?” said Cook.
Articulating all of those reasons, and communicating across multiple groups, moves you to a place where you can get a better handle on a set of shared goals. Even if it's just from the point of view of, ‘Now I understand why it is you think that thing over there is important; I can embrace that now as something useful for the entire organization, instead of just thinking your goals might interfere with my goals.’
“It’s definitely a ‘go slow to go fast’ kind of thing,” said Cook. “Otherwise, people start to push back because they don't understand what's going on.”
Making decisions with imperfect data
There are some organizations that assume data is perfect, and they need perfect data. You can get perfect data, but it comes at a cost: the time, effort and energy needed to perfect the data may render it obsolete before it gets applied, and at some point, you reach diminishing returns on the effort. Therefore, we live in the reality that perfect data is a myth because it cannot be achieved efficiently within the time you have.
Uddin encouraged the audience to consider the types of data they have and the decisions they are making. Financial data tends to be very good because of the strict controls on processes and systems; operational data varies widely in quality and variety, especially as it has become easier to capture. He presented a basic framework to think about working with imperfect data driven by the implications of the decision itself.
- High materiality decisions (the Y axis) require either high-quality data (with corresponding investment) or rules guiding usage and limitations.
- Low materiality decisions have a lower standard, and you can get by with directionally correct or good enough.
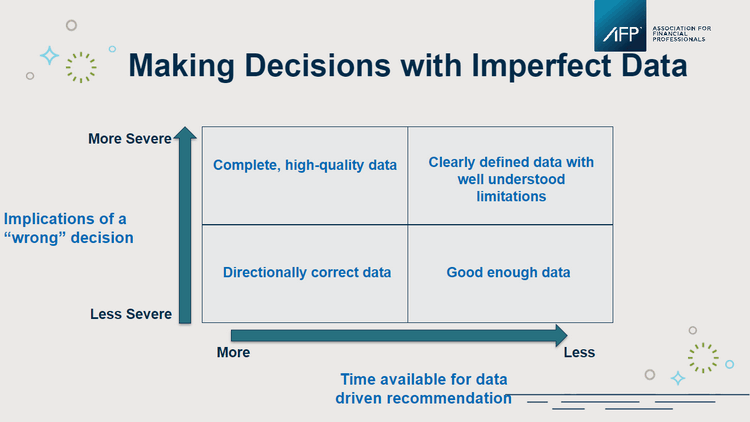
The need for accuracy in data comes down to how it's being used. For example, 80% accuracy on financial statements isn't going to get you through an audit; 80% accuracy on a wire transfer is not acceptable and the implications of getting those wrong are severe. Uddin said it’s important to break your data into three categories to help think about how to spend your time (the X axis of the matrix):
- Provable. You want to put in the effort to automate and operationalize these facts; there is not a lot of judgment, so you do the work.
- Quantifiable. Sometimes, the “data” in this category is really an assumption that can be stated numerically. Here, define the assumption and the sensitivity around the recommendation. If the percentage went from 5% to 10%, would I still do the same thing?
- Experience/judgments. We all bring business acumen to the table, and we’re paid for our experience and judgments, but we need to be more skeptical of decisions made based on judgments than ones made from provable data.
When evaluating the data in front of you, or a report based on that data, Uddin advises that you ask three critical questions about how it was compiled:
- What got excluded from this data? How did you treat data aberrations? Were they dropped or averaged into the overall results? That question tells you something about how it was collected. Sometimes you are matching different data sources; sometimes people take shortcuts to get to an answer they “believe” to be true.
- Did all the data sources indicate the same thing in this aggregation you just presented to me, or did you pick one over the other? Understand how it was developed and mashed together. For example, did you say ‘my sales data is better than my operational data, so I'm going to use more sales data in my aggregated values’?
- Do you believe that the data we're talking about here is complete, and do you think it represents the conclusions you've presented?
Notice that none of these questions elicit a yes or no answer. What you want to do is have a dialogue about whether you can trust the data for the type of decision you need to make.
What does finance get wrong when they're trying to get their data right? “Finance often has too much faith in the models and the numbers,” said Uddin. “Spreadsheet models look scientific, but just because something is in a spreadsheet format, doesn’t mean it’s provable. We all need to be a little skeptical.”
Want to learn more about this topic? Read part two of this two-part article. Are you ready to get your data right? Check out the AFP FP&A Guide: Get Your Data Right.
Copyright © 2024 Association for Financial Professionals, Inc.
All rights reserved.