Articles
9 Critical Pitfalls to Avoid on the AI Journey in Finance
- By Ashok Manthena, MBA
- Published: 7/22/2022
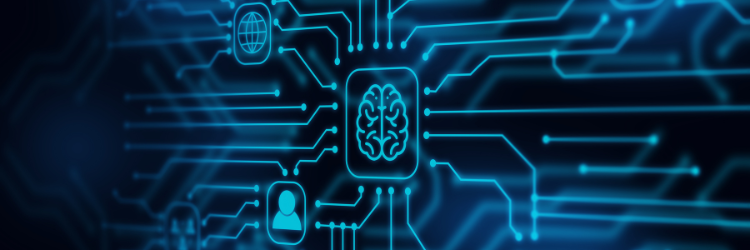
Data insights provided by AI can allow finance professionals to move faster and nimbler, make more data-driven decisions and transform business operations. The main applications of AI in finance are financial modeling and forecasting.
Traditionally, finance professionals have used Excel for modeling, but that Excel tends to produce inaccurate results because it overgeneralizes the relationship between several data elements.
AI, on the other hand, can develop a formula based on previous data trends that can be combined with future-related assumptions to improve modeling outcomes. It can also help finance teams increase their efficiency and effectiveness in the wake of resource shortages caused by the pandemic.
Businesses can get an edge over the competition by becoming early adopters, but AI applications can only be as successful as the data and processes used with them. By understanding the potential and constraints of AI, finance professionals can better manage AI applications and reduce their risks. Here are nine critical pitfalls to avoid when embarking on the AI journey in finance:
DATA PITFALLS
1. Lack of Historical Data
AI models need a sufficient amount of data to learn the correlations between different data points. As granularity increases, the models need more years of data. However, due to a tax audit requirement, companies purge some data after seven years. Companies also delete data during system updates because maintaining historical data is expensive and requires upkeep.
A lack of historical data will negatively impact an AI model’s accuracy, as well as the number of future periods for which finance professionals can forecast. Ultimately, finance needs to determine which data is relevant and assume the cost associated with storing it.
2. Poor Data Quality
Poor data quality will lead to issues during AI implementation. Things that can affect the data quality include missing data for chart of accounts (COA) members, late entries, top-down adjustments and accruals. Additionally, COA hierarchy changes during divestitures and acquisitions affect the statistical properties of the dataset.
3. Sparse Data
Finance has a data sparsity problem. When there are several COA members with zeros or missing values, the dataset is considered sparse, which presents two challenges: 1) AI models still have to perform all the calculations for these missing values, using up precious processing resources, and 2) sparse datasets also affect the efficacy and precision of the outcomes.
4. Poor Curation of Training Data
Best practice is to manually extract and prepare data to feed AI models in the early phases of AI implementation. However, a lack of attention to detail during manual processing can result in data mistakes. Variation in data processing from month to month may cause the AI models' training to deviate, which will lead to erroneous output.
5. Data Silos
Data silos are places where access to the data is restricted to a small number of people. Data silos develop as a result of data distribution across many source systems.
For example, those in finance have access to financial data in enterprise resource planning (ERP) systems. However, if they want to analyze inventory data or granular marketing data, they must rely on a different team to send it. This creates friction in experimentation and continuous improvement of the AI pipeline.
Data silos can also be created by organizational culture, system access or historical processes, all leading to the same result: preventing finance teams from having a comprehensive understanding of the business.
PROCESS PITFALLS
6. Accuracy as the Sole Metric to Measuring Efficacy
Accuracy is frequently chosen as a metric to gauge a model's efficacy. But the success of the project should not be judged solely on how accurately the forecasts match the actuals. Instead, the model should be compared to actuals, manually forecasted data, and the naïve forecast (applying the previous period’s forecast to the current forecast without adjustment). If the AI forecasted data is closer to actuals than the manual and naïve forecast, then it should be taken as a win. Combining this with the time savings will create enormous business value for finance functions.
7. Premature Automation
It would be a recipe for failure to invest in automating data extracts, feeds and the building of data warehouses before determining the right use case where AI can be deployed effectively. The goal should be to use AI to generate meaningful outcomes that support data-driven decision-making. Moreover, AI model building and deploying is relatively cheaper than building data warehouses and automating integrations. So, automation should be carried out once the AI path is determined and proven.
8. Lack of Awareness of AI’s Limitations
Not all financial use cases lend themselves well to AI modeling. Even with world class AI models and software, the output of AI depends on the signal in data and having the right drivers. Unreasonable standards of efficacy for an AI pipeline prevent finance professionals from benefiting from incremental improvements in data-driven decision making.
Preliminary training in data science and machine learning (ML) will help finance professionals understand how AI and ML work and how to reap the benefits from them.
9. No Room for Experimentation
Because AI is still in its beginning stages in finance, there is a great deal of potential for trial and error until the data points and procedures can be codified. Unlike an ERP configuration, AI cannot currently be applied in a “big bang” manner. The availability of the data, manual modeling efforts and predictability of the data should all be taken into consideration when choosing use cases.
An experimental method to deploy AI could generate better outcomes than a waterfall strategy, because the output of the early stages of AI will probably have a lot of room for improvement.
HOW AI CAN IMPROVE FORECASTING
AI transformation requires finance teams to think unconventionally and to continue to learn. Those that are able to implement AI successfully can reduce business risk through quicker, more effective decision-making.
Learn about the critical steps in the digital transformation process in the 2022 AFP FP&A Guide: Get Your Data Right.
Copyright © 2024 Association for Financial Professionals, Inc.
All rights reserved.