Articles
Applying AI to an EPM System
- By John Behme, CEO of Analytic View, former Director of Global Financial Systems at a global manufacturer
- Published: 8/20/2024
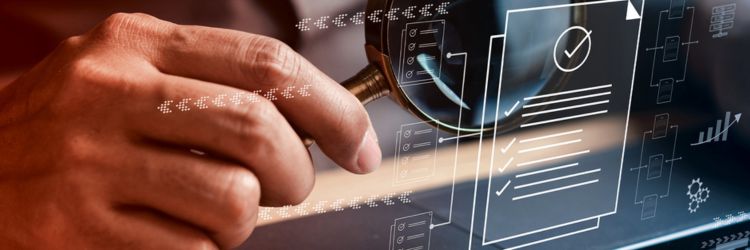
I worked at a publicly traded manufacturer headquartered in the Southeastern United States, with under $10B in revenue annually and operations in 30+ countries. Half the business is consumer packaging for goods you find in your cupboard; the other half includes industrial products, like the giant reels that hold big fiber optic cables or the packaging that protects your washer and dryer, in addition to some unique packaging in specific industry segments.
My role was to support both the Controllership and FP&A functions in most things related to financial systems. We had quite a few projects going on in the enterprise performance management space, so that had been my main focus for the last few years. Like many companies, we struggled with forecast accuracy, so that was the hot area at the time. The company is a volume business, so if we could produce an accurate demand forecast, we could drive an accurate top-line revenue number, as well as the remainder of the P&L. Equally important was the significant time savings our FP&A organization would gain by using machine learning technology.
From Pilot Test to Production
Our team knew we needed to be able to forecast more accurately. We knew our EPM tool well because we used it for many things we did in our monthly cycles, including global consolidations, budgeting, forecasting, account reconciliations and tax provisioning. Using it for machine learning capabilities seemed like a natural extension.
We started simple, working with one plant that had a great data set that we could leverage, a small number of product lines and great teammates. We kept this effort within the four walls of finance and moved quickly with a handful of people.
We were able to project purchasing demand about 25% more accurately! We got pretty excited when we considered extrapolating that benefit across our hundreds of global plants and the possibility of creating more accurate guidance on the top line down to operating profit, as well as better working capital management through lower inventory. These improvements also had the possibility to bring focus and insight to key metrics that the management team incentivized to focus on, so needless to say, it is pretty important! We could also see producing more forecasts with fewer FTE hours and having people do better work instead of grinding on forecasts.
Our CFO gave us the green light to start this journey and also believed that FP&A should own these tools. He wanted FP&A to drive the business and stick our nose in the middle of the sales and operations process (S&OP) within our businesses.
The second stage was to deploy the tool to 14 plants in Europe. We were cutting time out of the demand planning process, standardizing it and applying it to an SKU level. We were also working with another one of our U.S.-based businesses on a similar process, as they had really struggled with accuracy in the last year. We believed we would be able to turn these projects with our businesses very quickly, at 12 weeks per business unit, once we got up to full speed.
Data
The data we were using mostly resided in our ERP systems or data warehouse; however, the tool could quickly ingest the data from numerous sources, both internal and external. It could also analyze the data across a number of parameters, looking at things like zero values, sparsity — to the extent that there are deficiencies in the data — or quality deficiencies that imply a need to enrich the data set further.
People
We did not have any data scientists working with us, nor did our people need to be data scientists; they had to understand why a specific model was chosen for a specific product and what was driving that product's forecast.
My team spent four days with the EPM team in a boot camp, just understanding data sets and models and how to enrich data. We were able to apply features (ex. interest rates, paper prices, customer stock prices etc.) and events (ex. plant shutdowns, holidays, sporting events, etc.) that we believed may be impacting our business, as well as our customers’ businesses. This became a sort of “trial and error” game to see what you could throw at the models and what had an impact and what did not. Being able to train models and see results quickly was one of the key capabilities of the tool. We called it the “democratization of data science” and our FP&A teams could model those scenarios.
Leveraging our existing EPM platform allowed us to move so much faster. We could still work in our customary environment with familiar normal hierarchies, but we could also connect to additional rich data sets within the platform, including forms-based, Excel-based, PowerPoint presentations or Word documents.
Process
To be fully transparent, our budgeting and forecasting processes were very archaic. We spent a ton of time grinding through spreadsheets and loading them into our EPM tool, and while some of our businesses did some driver-based work, it was sporadic. We saw machine learning as a chance for us to jumpstart our transformation and put data scientists' tools in the finance organization's hands.
Explainability and transparency were critical factors for adoption and were built into our process. It's indefensible to walk into a meeting and simply say that these are the results produced by machine learning. People will just not trust it. So, let’s say a demand forecast shows we will sell 100 units; the software may create the crosswalk (or waterfall chart) that shows the historical data demand, which indicates 93 units of demand. Other factors, such as the Federal Reserve economic data, interest rates, unemployment, population growth, or maybe a promotion or price reduction, are adding to or taking away, which explains how we're getting to those hundred units. This is just not core information that tools have been able to provide in the past.
Technology
I think EPM (or CPM) needs to be at the forefront of your transformation. It houses your external reporting, management reporting and control environment reporting. I think the forecasting side is more important to senior leadership than the controllership side, so that is where you want to place your most sophisticated technology assets, such as machine learning and AI.
If you truly are doing a transformation, don’t put the effort into a detailed forecasting process and then turn around and have machine learning come back with better answers. Start with AI and try to skip some of that grinding work.
AFP FP&A Guide to AI-Powered Finance
Get more on this topic. Whether you're just beginning to explore AI's potential or seeking a clear path forward, this guide offers practical steps to help you confidently embark on your AI journey.
Copyright © 2024 Association for Financial Professionals, Inc.
All rights reserved.